How a $300K Bored Ape Yacht Club NFT was accidentally sold for $3K
The Bored Ape Yacht Club is one of the most prestigious NFT collections in the world. A collection of 10,000 NFTs, each depicting an ape with different traits and visual attributes, Jimmy Fallon, Steph Curry and Post Malone are among their star-studded owners. Right now the price of entry is 52 ether, or $210,000.
Which is why it's so painful to see that someone accidentally sold their Bored Ape NFT for $3,066.
Unusual trades are often a sign of funny business, as in the case of the person who spent $530 million to buy an NFT from themselves. In Saturday's case, the cause was a simple, devastating "fat-finger error." That's when people make a trade online for the wrong thing, or for the wrong amount. Here the owner, real name Max or username maxnaut, meant to list his Bored Ape for 75 ether, or around $300,000. Instead he accidentally listed it for 0.75. One hundredth the intended price.
It was bought instantaneously. The buyer paid an extra $34,000 to speed up the transaction, ensuring no one could snap it up before them. The Bored Ape was then promptly listed for $248,000. The transaction appears to have been done by a bot, which can be coded to immediately buy NFTs listed below a certain price on behalf of their owners in order to take advantage of these exact situations.
"How'd it happen? A lapse of concentration I guess," Max told me. "I list a lot of items every day and just wasn't paying attention properly. I instantly saw the error as my finger clicked the mouse but a bot sent a transaction with over 8 eth [$34,000] of gas fees so it was instantly sniped before I could click cancel, and just like that, $250k was gone."
"And here within the beauty of the Blockchain you can see that it is both honest and unforgiving," he added.
Fat finger trades happen sporadically in traditional finance -- like the Japanese trader who almost bought 57% of Toyota's stock in 2014 -- but most financial institutions will stop those transactions if alerted quickly enough. Since cryptocurrency and NFTs are designed to be decentralized, you essentially have to rely on the goodwill of the buyer to reverse the transaction.
Fat finger errors in cryptocurrency trades have made many a headline over the past few years. Back in 2019, the company behind Tether, a cryptocurrency pegged to the US dollar, nearly doubled its own coin supply when it accidentally created $5 billion-worth of new coins. In March, BlockFi meant to send 700 Gemini Dollars to a set of customers, worth roughly $1 each, but mistakenly sent out millions of dollars worth of bitcoin instead. Last month a company erroneously paid a $24 million fee on a $100,000 transaction.
Similar incidents are increasingly being seen in NFTs, now that many collections have accumulated in market value over the past year. Last month someone tried selling a CryptoPunk NFT for $19 million, but accidentally listed it for $19,000 instead. Back in August, someone fat finger listed their Bored Ape for $26,000, an error that someone else immediately capitalized on. The original owner offered $50,000 to the buyer to return the Bored Ape -- but instead the opportunistic buyer sold it for the then-market price of $150,000.
"The industry is so new, bad things are going to happen whether it's your fault or the tech," Max said. "Once you no longer have control of the outcome, forget and move on."
The Bored Ape Yacht Club launched back in April 2021, with 10,000 NFTs being sold for 0.08 ether each -- about $190 at the time. While NFTs are often associated with individual digital art pieces, collections like the Bored Ape Yacht Club, which allow owners to flaunt their NFTs by using them as profile pictures on social media, are becoming increasingly prevalent. The Bored Ape Yacht Club has since become the second biggest NFT collection in the world, second only to CryptoPunks, which launched in 2017 and is considered the "original" NFT collection.
More on Web3 & Crypto

Vitalik
2 years ago
An approximate introduction to how zk-SNARKs are possible (part 2)
If tasked with the problem of coming up with a zk-SNARK protocol, many people would make their way to this point and then get stuck and give up. How can a verifier possibly check every single piece of the computation, without looking at each piece of the computation individually? But it turns out that there is a clever solution.
Polynomials
Polynomials are a special class of algebraic expressions of the form:
- x+5
- x^4
- x^3+3x^2+3x+1
- 628x^{271}+318x^{270}+530x^{269}+…+69x+381
i.e. they are a sum of any (finite!) number of terms of the form cx^k
There are many things that are fascinating about polynomials. But here we are going to zoom in on a particular one: polynomials are a single mathematical object that can contain an unbounded amount of information (think of them as a list of integers and this is obvious). The fourth example above contained 816 digits of tau, and one can easily imagine a polynomial that contains far more.
Furthermore, a single equation between polynomials can represent an unbounded number of equations between numbers. For example, consider the equation A(x)+ B(x) = C(x). If this equation is true, then it's also true that:
- A(0)+B(0)=C(0)
- A(1)+B(1)=C(1)
- A(2)+B(2)=C(2)
- A(3)+B(3)=C(3)
And so on for every possible coordinate. You can even construct polynomials to deliberately represent sets of numbers so you can check many equations all at once. For example, suppose that you wanted to check:
- 12+1=13
- 10+8=18
- 15+8=23
- 15+13=28
You can use a procedure called Lagrange interpolation to construct polynomials A(x) that give (12,10,15,15) as outputs at some specific set of coordinates (eg. (0,1,2,3)), B(x) the outputs (1,8,8,13) on thos same coordinates, and so forth. In fact, here are the polynomials:
- A(x)=-2x^3+\frac{19}{2}x^2-\frac{19}{2}x+12
- B(x)=2x^3-\frac{19}{2}x^2+\frac{29}{2}x+1
- C(x)=5x+13
Checking the equation A(x)+B(x)=C(x) with these polynomials checks all four above equations at the same time.
Comparing a polynomial to itself
You can even check relationships between a large number of adjacent evaluations of the same polynomial using a simple polynomial equation. This is slightly more advanced. Suppose that you want to check that, for a given polynomial F, F(x+2)=F(x)+F(x+1) with the integer range {0,1…89} (so if you also check F(0)=F(1)=1, then F(100) would be the 100th Fibonacci number)
As polynomials, F(x+2)-F(x+1)-F(x) would not be exactly zero, as it could give arbitrary answers outside the range x={0,1…98}. But we can do something clever. In general, there is a rule that if a polynomial P is zero across some set S=\{x_1,x_2…x_n\} then it can be expressed as P(x)=Z(x)*H(x), where Z(x)=(x-x_1)*(x-x_2)*…*(x-x_n) and H(x) is also a polynomial. In other words, any polynomial that equals zero across some set is a (polynomial) multiple of the simplest (lowest-degree) polynomial that equals zero across that same set.
Why is this the case? It is a nice corollary of polynomial long division: the factor theorem. We know that, when dividing P(x) by Z(x), we will get a quotient Q(x) and a remainder R(x) is strictly less than that of Z(x). Since we know that P is zero on all of S, it means that R has to be zero on all of S as well. So we can simply compute R(x) via polynomial interpolation, since it's a polynomial of degree at most n-1 and we know n values (the zeros at S). Interpolating a polynomial with all zeroes gives the zero polynomial, thus R(x)=0 and H(x)=Q(x).
Going back to our example, if we have a polynomial F that encodes Fibonacci numbers (so F(x+2)=F(x)+F(x+1) across x=\{0,1…98\}), then I can convince you that F actually satisfies this condition by proving that the polynomial P(x)=F(x+2)-F(x+1)-F(x) is zero over that range, by giving you the quotient:
H(x)=\frac{F(x+2)-F(x+1)-F(x)}{Z(x)}
Where Z(x) = (x-0)*(x-1)*…*(x-98).
You can calculate Z(x) yourself (ideally you would have it precomputed), check the equation, and if the check passes then F(x) satisfies the condition!
Now, step back and notice what we did here. We converted a 100-step-long computation into a single equation with polynomials. Of course, proving the N'th Fibonacci number is not an especially useful task, especially since Fibonacci numbers have a closed form. But you can use exactly the same basic technique, just with some extra polynomials and some more complicated equations, to encode arbitrary computations with an arbitrarily large number of steps.
see part 3

Ben
2 years ago
The Real Value of Carbon Credit (Climate Coin Investment)
Disclaimer : This is not financial advice for any investment.
TL;DR
You might not have realized it, but as we move toward net zero carbon emissions, the globe is already at war.
According to the Paris Agreement of COP26, 64% of nations have already declared net zero, and the issue of carbon reduction has already become so important for businesses that it affects their ability to survive. Furthermore, the time when carbon emission standards will be defined and controlled on an individual basis is becoming closer.
Since 2017, the market for carbon credits has experienced extraordinary expansion as a result of widespread talks about carbon credits. The carbon credit market is predicted to expand much more once net zero is implemented and carbon emission rules inevitably tighten.
Hello! Ben here from Nonce Classic. Nonce Classic has recently confirmed the tremendous growth potential of the carbon credit market in the midst of a major trend towards the global goal of net zero (carbon emissions caused by humans — carbon reduction by humans = 0 ). Moreover, we too believed that the questions and issues the carbon credit market suffered from the last 30–40yrs could be perfectly answered through crypto technology and that is why we have added a carbon credit crypto project to the Nonce Classic portfolio. There have been many teams out there that have tried to solve environmental problems through crypto but very few that have measurable experience working in the carbon credit scene. Thus we have put in our efforts to find projects that are not crypto projects created for the sake of issuing tokens but projects that pragmatically use crypto technology to combat climate change by solving problems of the current carbon credit market. In that process, we came to hear of Climate Coin, a veritable carbon credit crypto project, and us Nonce Classic as an accelerator, have begun contributing to its growth and invested in its tokens. Starting with this article, we plan to publish a series of articles explaining why the carbon credit market is bullish, why we invested in Climate Coin, and what kind of project Climate Coin is specifically. In this first article let us understand the carbon credit market and look into its growth potential! Let’s begin :)
The Unavoidable Entry of the Net Zero Era
Net zero means... Human carbon emissions are balanced by carbon reduction efforts. A non-environmentalist may find it hard to accept that net zero is attainable by 2050. Global cooperation to save the earth is happening faster than we imagine.
In the Paris Agreement of COP26, concluded in Glasgow, UK on Oct. 31, 2021, nations pledged to reduce worldwide yearly greenhouse gas emissions by more than 50% by 2030 and attain net zero by 2050. Governments throughout the world have pledged net zero at the national level and are holding each other accountable by submitting Nationally Determined Contributions (NDC) every five years to assess implementation. 127 of 198 nations have declared net zero.
Each country's 1.5-degree reduction plans have led to carbon reduction obligations for companies. In places with the strictest environmental regulations, like the EU, companies often face bankruptcy because the cost of buying carbon credits to meet their carbon allowances exceeds their operating profits. In this day and age, minimizing carbon emissions and securing carbon credits are crucial.
Recent SEC actions on climate change may increase companies' concerns about reducing emissions. The SEC required all U.S. stock market companies to disclose their annual greenhouse gas emissions and climate change impact on March 21, 2022. The SEC prepared the proposed regulation through in-depth analysis and stakeholder input since last year. Three out of four SEC members agreed that it should pass without major changes. If the regulation passes, it will affect not only US companies, but also countless companies around the world, directly or indirectly.
Even companies not listed on the U.S. stock market will be affected and, in most cases, required to disclose emissions. Companies listed on the U.S. stock market with significant greenhouse gas emissions or specific targets are subject to stricter emission standards (Scope 3) and disclosure obligations, which will magnify investigations into all related companies. Greenhouse gas emissions can be calculated three ways. Scope 1 measures carbon emissions from a company's facilities and transportation. Scope 2 measures carbon emissions from energy purchases. Scope 3 covers all indirect emissions from a company's value chains.
The SEC's proposed carbon emission disclosure mandate and regulations are one example of how carbon credit policies can cross borders and affect all parties. As such incidents will continue throughout the implementation of net zero, even companies that are not immediately obligated to disclose their carbon emissions must be prepared to respond to changes in carbon emission laws and policies.
Carbon reduction obligations will soon become individual. Individual consumption has increased dramatically with improved quality of life and convenience, despite national and corporate efforts to reduce carbon emissions. Since consumption is directly related to carbon emissions, increasing consumption increases carbon emissions. Countries around the world have agreed that to achieve net zero, carbon emissions must be reduced on an individual level. Solutions to individual carbon reduction are being actively discussed and studied under the term Personal Carbon Trading (PCT).
PCT is a system that allows individuals to trade carbon emission quotas in the form of carbon credits. Individuals who emit more carbon than their allotment can buy carbon credits from those who emit less. European cities with well-established carbon credit markets are preparing for net zero by conducting early carbon reduction prototype projects. The era of checking product labels for carbon footprints, choosing low-emissions transportation, and worrying about hot shower emissions is closer than we think.
The Market for Carbon Credits Is Expanding Fearfully
Compliance and voluntary carbon markets make up the carbon credit market.
A Compliance Market enforces carbon emission allowances for actors. Companies in industries that previously emitted a lot of carbon are included in the mandatory carbon market, and each government receives carbon credits each year. If a company's emissions are less than the assigned cap and it has extra carbon credits, it can sell them to other companies that have larger emissions and require them (Cap and Trade). The annual number of free emission permits provided to companies is designed to decline, therefore companies' desire for carbon credits will increase. The compliance market's yearly trading volume will exceed $261B in 2020, five times its 2017 level.
In the Voluntary Market, carbon reduction is voluntary and carbon credits are sold for personal reasons or to build market participants' eco-friendly reputations. Even if not in the compliance market, it is typical for a corporation to be obliged to offset its carbon emissions by acquiring voluntary carbon credits. When a company seeks government or company investment, it may be denied because it is not net zero. If a significant shareholder declares net zero, the companies below it must execute it. As the world moves toward ESG management, becoming an eco-friendly company is no longer a strategic choice to gain a competitive edge, but an important precaution to not fall behind. Due to this eco-friendly trend, the annual market volume of voluntary emission credits will approach $1B by November 2021. The voluntary credit market is anticipated to reach $5B to $50B by 2030. (TSCVM 2021 Report)
In conclusion
This article analyzed how net zero, a target promised by countries around the world to combat climate change, has brought governmental, corporate, and human changes. We discussed how these shifts will become more obvious as we approach net zero, and how the carbon credit market would increase exponentially in response. In the following piece, let's analyze the hurdles impeding the carbon credit market's growth, how the project we invested in tries to tackle these issues, and why we chose Climate Coin. Wait! Jim Skea, co-chair of the IPCC working group, said,
“It’s now or never, if we want to limit global warming to 1.5°C” — Jim Skea
Join nonceClassic’s community:
Telegram: https://t.me/non_stock
Youtube: https://www.youtube.com/channel/UCqeaLwkZbEfsX35xhnLU2VA
Twitter: @nonceclassic
Mail us : general@nonceclassic.org
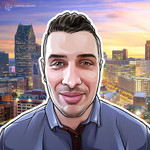
Sam Bourgi
2 years ago
DAOs are legal entities in Marshall Islands.
The Pacific island state recognizes decentralized autonomous organizations.
The Republic of the Marshall Islands has recognized decentralized autonomous organizations (DAOs) as legal entities, giving collectively owned and managed blockchain projects global recognition.
The Marshall Islands' amended the Non-Profit Entities Act 2021 that now recognizes DAOs, which are blockchain-based entities governed by self-organizing communities. Incorporating Admiralty LLC, the island country's first DAO, was made possible thanks to the amendement. MIDAO Directory Services Inc., a domestic organization established to assist DAOs in the Marshall Islands, assisted in the incorporation.
The new law currently allows any DAO to register and operate in the Marshall Islands.
“This is a unique moment to lead,” said Bobby Muller, former Marshall Islands chief secretary and co-founder of MIDAO. He believes DAOs will help create “more efficient and less hierarchical” organizations.
A global hub for DAOs, the Marshall Islands hopes to become a global hub for DAO registration, domicile, use cases, and mass adoption. He added:
"This includes low-cost incorporation, a supportive government with internationally recognized courts, and a technologically open environment."
According to the World Bank, the Marshall Islands is an independent island state in the Pacific Ocean near the Equator. To create a blockchain-based cryptocurrency that would be legal tender alongside the US dollar, the island state has been actively exploring use cases for digital assets since at least 2018.
In February 2018, the Marshall Islands approved the creation of a new cryptocurrency, Sovereign (SOV). As expected, the IMF has criticized the plan, citing concerns that a digital sovereign currency would jeopardize the state's financial stability. They have also criticized El Salvador, the first country to recognize Bitcoin (BTC) as legal tender.
Marshall Islands senator David Paul said the DAO legislation does not pose the same issues as a government-backed cryptocurrency. “A sovereign digital currency is financial and raises concerns about money laundering,” . This is more about giving DAOs legal recognition to make their case to regulators, investors, and consumers.
You might also like

Sam Warain
2 years ago
The Brilliant Idea Behind Kim Kardashian's New Private Equity Fund
Kim Kardashian created Skky Partners. Consumer products, internet & e-commerce, consumer media, hospitality, and luxury are company targets.
Some call this another Kardashian publicity gimmick.
This maneuver is brilliance upon closer inspection. Why?
1) Kim has amassed a sizable social media fan base:
Over 320 million Instagram and 70 million Twitter users follow Kim Kardashian.
Kim Kardashian's Instagram account ranks 8th. Three Kardashians in top 10 is ridiculous.
This gives her access to consumer data. She knows what people are discussing. Investment firms need this data.
Quality, not quantity, of her followers matters. Studies suggest that her following are more engaged than Selena Gomez and Beyonce's.
Kim's followers are worth roughly $500 million to her brand, according to a research. They trust her and buy what she recommends.
2) She has a special aptitude for identifying trends.
Kim Kardashian can sense trends.
She's always ahead of fashion and beauty trends. She's always trying new things, too. She doesn't mind making mistakes when trying anything new. Her desire to experiment makes her a good business prospector.
Kim has also created a lifestyle brand that followers love. Kim is an entrepreneur, mom, and role model, not just a reality TV star or model. She's established a brand around her appearance, so people want to buy her things.
Her fragrance collection has sold over $100 million since its 2009 introduction, and her Sears apparel line did over $200 million in its first year.
SKIMS is her latest $3.2bn brand. She can establish multibillion-dollar firms with her enormous distribution platform.
Early founders would kill for Kim Kardashian's network.
Making great products is hard, but distribution is more difficult. — David Sacks, All-in-Podcast
3) She can delegate the financial choices to Jay Sammons, one of the greatest in the industry.
Jay Sammons is well-suited to develop Kim Kardashian's new private equity fund.
Sammons has 16 years of consumer investing experience at Carlyle. This will help Kardashian invest in consumer-facing enterprises.
Sammons has invested in Supreme and Beats Electronics, both of which have grown significantly. Sammons' track record and competence make him the obvious choice.
Kim Kardashian and Jay Sammons have joined forces to create a new business endeavor. The agreement will increase Kardashian's commercial empire. Sammons can leverage one of the world's most famous celebrities.
“Together we hope to leverage our complementary expertise to build the next generation consumer and media private equity firm” — Kim Kardashian
Kim Kardashian is a successful businesswoman. She developed an empire by leveraging social media to connect with fans. By developing a global lifestyle brand, she has sold things and experiences that have made her one of the world's richest celebrities.
She's a shrewd entrepreneur who knows how to maximize on herself and her image.
Imagine how much interest Kim K will bring to private equity and venture capital.
I'm curious about the company's growth.

Rachel Greenberg
2 years ago
The Unsettling Fact VC-Backed Entrepreneurs Don't Want You to Know
What they'll do is scarier.
My acquaintance recently joined a VC-funded startup. Money, equity, and upside possibilities were nice, but he had a nagging dread.
They just secured a $40M round and are hiring like crazy to prepare for their IPO in two years. All signals pointed to this startup's (a B2B IT business in a stable industry) success, and its equity-holding workers wouldn't pass that up.
Five months after starting the work, my friend struggled with leaving. We might overlook the awful culture and long hours at the proper price. This price plus the company's fate and survival abilities sent my friend departing in an unpleasant unplanned resignation before jumping on yet another sinking ship.
This affects founders. This affects VC-backed companies (and all businesses). This affects anyone starting, buying, or running a business.
Here's the under-the-table approach that's draining VC capital, leaving staff terrified (or jobless), founders rattled, and investors upset. How to recognize, solve, and avoid it
The unsettling reality behind door #1
You can't raise money off just your looks, right? If "looks" means your founding team's expertise, then maybe. In my friend's case, the founding team's strong qualifications and track records won over investors before talking figures.
They're hardly the only startup to raise money without a profitable customer acquisition strategy. Another firm raised money for an expensive sleep product because it's eco-friendly. They were off to the races with a few keywords and key players.
Both companies, along with numerous others, elected to invest on product development first. Company A employed all the tech, then courted half their market (they’re a tech marketplace that connects two parties). Company B spent millions on R&D to create a palatable product, then flooded the world with marketing.
My friend is on Company B's financial team, and he's seen where they've gone wrong. It's terrible.
Company A (tech market): Growing? Not quite. To achieve the ambitious expansion they (and their investors) demand, they've poured much of their little capital into salespeople: Cold-calling commission and salary salesmen. Is it working? Considering attrition and companies' dwindling capital, I don't think so.
Company B (green sleep) has been hiring, digital marketing, and opening new stores like crazy. Growing expenses should result in growing revenues and a favorable return on investment; if you grow too rapidly, you may neglect to check that ROI.
Once Company A cut headcount and Company B declared “going concerned”, my friend realized both startups had the same ailment and didn't recognize it.
I shouldn't have to ask a friend to verify a company's cash reserves and profitability to spot a financial problem. It happened anyhow.
The frightening part isn't that investors were willing to invest millions without product-market fit, CAC, or LTV estimates. That's alarming, but not as scary as the fact that startups aren't understanding the problem until VC rounds have dried up.
When they question consultants if their company will be around in 6 months. It’s a red flag. How will they stretch $20M through a 2-year recession with a $3M/month burn rate and no profitability? Alarms go off.
Who's in danger?
In a word, everyone who raised money without a profitable client acquisition strategy or enough resources to ride out dry spells.
Money mismanagement and poor priorities affect every industry (like sinking all your capital into your product, team, or tech, at the expense of probing what customer acquisition really takes and looks like).
This isn't about tech, real estate, or recession-proof luxury products. Fast, cheap, easy money flows into flashy-looking teams with buzzwords, trending industries, and attractive credentials.
If these companies can't show progress or get a profitable CAC, they can't raise more money. They die if they can't raise more money (or slash headcount and find shoestring budget solutions until they solve the real problem).
The kiss of death (and how to avoid it)
If you're running a startup and think raising VC is the answer, pause and evaluate. Do you need the money now?
I'm not saying VC is terrible or has no role. Founders have used it as a Band-Aid for larger, pervasive problems. Venture cash isn't a crutch for recruiting consumers profitably; it's rocket fuel to get you what and who you need.
Pay-to-play isn't a way to throw money at the wall and hope for a return. Pay-to-play works until you run out of money, and if you haven't mastered client acquisition, your cash will diminish quickly.
How can you avoid this bottomless pit? Tips:
Understand your burn rate
Keep an eye on your growth or profitability.
Analyze each and every marketing channel and initiative.
Make lucrative customer acquisition strategies and satisfied customers your top two priorities. not brand-new products. not stellar hires. avoid the fundraising rollercoaster to save time. If you succeed in these two tasks, investors will approach you with their thirsty offers rather than the other way around, and your cash reserves won't diminish as a result.
Not as much as your grandfather
My family friend always justified expensive, impractical expenditures by saying it was only monopoly money. In business, startups, and especially with money from investors expecting a return, that's not true.
More founders could understand that there isn't always another round if they viewed VC money as their own limited pool. When the well runs dry, you must refill it or save the day.
Venture financing isn't your grandpa's money. A discerning investor has entrusted you with dry powder in the hope that you'll use it wisely, strategically, and thoughtfully. Use it well.

Tim Denning
2 years ago
Elon Musk’s Rich Life Is a Nightmare
I'm sure you haven't read about Elon's other side.
Elon divorced badly.
Nobody's surprised.
Imagine you're a parent. Someone isn't home year-round. What's next?
That’s what happened to YOLO Elon.
He can do anything. He can intervene in wars, shoot his mouth off, bang anyone he wants, avoid tax, make cool tech, buy anything his ego desires, and live anywhere exotic.
Few know his billionaire backstory. I'll tell you so you don't worship his lifestyle. It’s a cult.
Only his career succeeds. His life is a nightmare otherwise.
Psychopaths' schedule
Elon has said he works 120-hour weeks.
As he told the reporter about his job, he choked up, which was unusual for him.
His crazy workload and lack of sleep forced him to scold innocent Wall Street analysts. Later, he apologized.
In the same interview, he admits he hadn't taken more than a week off since 2001, when he was bedridden with malaria. Elon stays home after a near-death experience.
He's rarely outside.
Elon says he sometimes works 3 or 4 days straight.
He admits his crazy work schedule has cost him time with his kids and friends.
Elon's a slave
Elon's birthday description made him emotional.
Elon worked his entire birthday.
"No friends, nothing," he said, stuttering.
His brother's wedding in Catalonia was 48 hours after his birthday. That meant flying there from Tesla's factory prison.
He arrived two hours before the big moment, barely enough time to eat and change, let alone see his brother.
Elon had to leave after the bouquet was tossed to a crowd of billionaire lovers. He missed his brother's first dance with his wife.
Shocking.
He went straight to Tesla's prison.
The looming health crisis
Elon was asked if overworking affected his health.
Not great. Friends are worried.
Now you know why Elon tweets dumb things. Working so hard has probably caused him mental health issues.
Mental illness removed my reality filter. You do stupid things because you're tired.
Astronauts pelted Elon
Elon's overwork isn't the first time his life has made him emotional.
When asked about Neil Armstrong and Gene Cernan criticizing his SpaceX missions, he got emotional. Elon's heroes.
They're why he started the company, and they mocked his work. In another interview, we see how Elon’s business obsession has knifed him in the heart.
Once you have a company, you must feed, nurse, and care for it, even if it destroys you.
"Yep," Elon says, tearing up.
In the same interview, he's asked how Tesla survived the 2008 recession. Elon stopped the interview because he was crying. When Tesla and SpaceX filed for bankruptcy in 2008, he nearly had a nervous breakdown. He called them his "children."
All the time, he's risking everything.
Jack Raines explains best:
Too much money makes you a slave to your net worth.
Elon's emotions are admirable. It's one of the few times he seems human, not like an alien Cyborg.
Stop idealizing Elon's lifestyle
Building a side business that becomes a billion-dollar unicorn startup is a nightmare.
"Billionaire" means financially wealthy but otherwise broke. A rich life includes more than business and money.
This post is a summary. Read full article here