More on Entrepreneurship/Creators
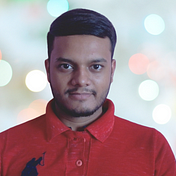
Sanjay Priyadarshi
1 year ago
Meet a Programmer Who Turned Down Microsoft's $10,000,000,000 Acquisition Offer
Failures inspire young developers
Jason citron created many products.
These products flopped.
Microsoft offered $10 billion for one of these products.
He rejected the offer since he was so confident in his success.
Let’s find out how he built a product that is currently valued at $15 billion.
Early in his youth, Jason began learning to code.
Jason's father taught him programming and IT.
His father wanted to help him earn money when he needed it.
Jason created video games and websites in high school.
Jason realized early on that his IT and programming skills could make him money.
Jason's parents misjudged his aptitude for programming.
Jason frequented online programming communities.
He looked for web developers. He created websites for those people.
His parents suspected Jason sold drugs online. When he said he used programming to make money, they were shocked.
They helped him set up a PayPal account.
Florida higher education to study video game creation
Jason never attended an expensive university.
He studied game design in Florida.
“Higher Education is an interesting part of society… When I work with people, the school they went to never comes up… only thing that matters is what can you do…At the end of the day, the beauty of silicon valley is that if you have a great idea and you can bring it to the life, you can convince a total stranger to give you money and join your project… This notion that you have to go to a great school didn’t end up being a thing for me.”
Jason's life was altered by Steve Jobs' keynote address.
After graduating, Jason joined an incubator.
Jason created a video-dating site first.
Bad idea.
Nobody wanted to use it when it was released, so they shut it down.
He made a multiplayer game.
It was released on Bebo. 10,000 people played it.
When Steve Jobs unveiled the Apple app store, he stopped playing.
The introduction of the app store resembled that of a new gaming console.
Jason's life altered after Steve Jobs' 2008 address.
“Whenever a new video game console is launched, that’s the opportunity for a new video game studio to get started, it’s because there aren’t too many games available…When a new PlayStation comes out, since it’s a new system, there’s only a handful of titles available… If you can be a launch title you can get a lot of distribution.”
Apple's app store provided a chance to start a video game company.
They released an app after 5 months of work.
Aurora Feint is the game.
Jason believed 1000 players in a week would be wonderful. A thousand players joined in the first hour.
Over time, Aurora Feints' game didn't gain traction. They don't make enough money to keep playing.
They could only make enough for one month.
Instead of buying video games, buy technology
Jason saw that they established a leaderboard, chat rooms, and multiplayer capabilities and believed other developers would want to use these.
They opted to sell the prior game's technology.
OpenFeint.
Assisting other game developers
They had no money in the bank to create everything needed to make the technology user-friendly.
Jason and Daniel designed a website saying:
“If you’re making a video game and want to have a drop in multiplayer support, you can use our system”
TechCrunch covered their website launch, and they gained a few hundred mailing list subscribers.
They raised seed funding with the mailing list.
Nearly all iPhone game developers started adopting the Open Feint logo.
“It was pretty wild… It was really like a whole social platform for people to play with their friends.”
What kind of a business model was it?
OpenFeint originally planned to make the software free for all games. As the game gained popularity, they demanded payment.
They later concluded it wasn't a good business concept.
It became free eventually.
Acquired for $104 million
Open Feint's users and employees grew tremendously.
GREE bought OpenFeint for $104 million in April 2011.
GREE initially committed to helping Jason and his team build a fantastic company.
Three or four months after the acquisition, Jason recognized they had a different vision.
He quit.
Jason's Original Vision for the iPad
Jason focused on distribution in 2012 to help businesses stand out.
The iPad market and user base were growing tremendously.
Jason said the iPad may replace mobile gadgets.
iPad gamers behaved differently than mobile gamers.
People sat longer and experienced more using an iPad.
“The idea I had was what if we built a gaming business that was more like traditional video games but played on tablets as opposed to some kind of mobile game that I’ve been doing before.”
Unexpected insight after researching the video game industry
Jason learned from studying the gaming industry that long-standing companies had advantages beyond a single release.
Previously, long-standing video game firms had their own distribution system. This distribution strategy could buffer time between successful titles.
Sony, Microsoft, and Valve all have gaming consoles and online stores.
So he built a distribution system.
He created a group chat app for gamers.
He envisioned a team-based multiplayer game with text and voice interaction.
His objective was to develop a communication network, release more games, and start a game distribution business.
Remaking the video game League of Legends
Jason and his crew reimagined a League of Legends game mode for 12-inch glass.
They adapted the game for tablets.
League of Legends was PC-only.
So they rebuilt it.
They overhauled the game and included native mobile experiences to stand out.
Hammer and Chisel was the company's name.
18 people worked on the game.
The game was funded. The game took 2.5 years to make.
Was the game a success?
July 2014 marked the game's release. The team's hopes were dashed.
Critics initially praised the game.
Initial installation was widespread.
The game failed.
As time passed, the team realized iPad gaming wouldn't increase much and mobile would win.
Jason was given a fresh idea by Stan Vishnevskiy.
Stan Vishnevskiy was a corporate engineer.
He told Jason about his plan to design a communication app without a game.
This concept seeded modern strife.
“The insight that he really had was to put a couple of dots together… we’re seeing our customers communicating around our own game with all these different apps and also ourselves when we’re playing on PC… We should solve that problem directly rather than needing to build a new game…we should start making it on PC.”
So began Discord.
Online socializing with pals was the newest trend.
Jason grew up playing video games with his friends.
He never played outside.
Jason had many great moments playing video games with his closest buddy, wife, and brother.
Discord was about providing a location for you and your group to speak and hang out.
Like a private cafe, bedroom, or living room.
Discord was developed for you and your friends on computers and phones.
You can quickly call your buddies during a game to conduct a conference call. Put the call on speaker and talk while playing.
Discord wanted to give every player a unique experience. Because coordinating across apps was a headache.
The entire team started concentrating on Discord.
Jason decided Hammer and Chisel would focus on their chat app.
Jason didn't want to make a video game.
How Discord attracted the appropriate attention
During the first five months, the entire team worked on the game and got feedback from friends.
This ensures product improvement. As a result, some teammates' buddies started utilizing Discord.
The team knew it would become something, but the result was buggy. App occasionally crashed.
Jason persuaded a gamer friend to write on Reddit about the software.
New people would find Discord. Why not?
Reddit users discovered Discord and 50 started using it frequently.
Discord was launched.
Rejecting the $10 billion acquisition proposal
Discord has increased in recent years.
It sends billions of messages.
Discord's users aren't tracked. They're privacy-focused.
Purchase offer
Covid boosted Discord's user base.
Weekly, billions of messages were transmitted.
Microsoft offered $10 billion for Discord in 2021.
Jason sold Open Feint for $104m in 2011.
This time, he believed in the product so much that he rejected Microsoft's offer.
“I was talking to some people in the team about which way we could go… The good thing was that most of the team wanted to continue building.”
Last time, Discord was valued at $15 billion.
Discord raised money on March 12, 2022.
The $15 billion corporation raised $500 million in 2021.

Stephen Moore
2 years ago
Adam Neumanns is working to create the future of living in a classic example of a guy failing upward.
The comeback tour continues…
First, he founded a $47 billion co-working company (sorry, a “tech company”).
He established WeLive to disrupt apartment life.
Then he created WeGrow, a school that tossed aside the usual curriculum to feed children's souls and release their potential.
He raised the world’s consciousness.
Then he blew it all up (without raising the world’s consciousness). (He bought a wave pool.)
Adam Neumann's WeWork business burned investors' money. The founder sailed off with unimaginable riches, leaving long-time employees with worthless stocks and the company bleeding money. His track record, which includes a failing baby clothing company, should have stopped investors cold.
Once the dust settled, folks went on. We forgot about the Neumanns! We forgot about the private jets, company retreats, many houses, and WeWork's crippling. In that moment, the prodigal son of entrepreneurship returned, choosing the blockchain as his industry. His homecoming tour began with Flowcarbon, which sold Goddess Nature Tokens to lessen companies' carbon footprints.
Did it work?
Of course not.
Despite receiving $70 million from Andreessen Horowitz's a16z, the project has been halted just two months after its announcement.
This triumph should lower his grade.
Neumann seems to have moved on and has another revolutionary idea for the future of living. Flow (not Flowcarbon) aims to help people live in flow and will launch in 2023. It's the classic Neumann pitch: lofty goals, yogababble, and charisma to attract investors.
It's a winning formula for one investment fund. a16z has backed the project with its largest single check, $350 million. It has a splash page and 3,000 rental units, but is valued at over $1 billion. The blog post praised Neumann for reimagining the office and leading a paradigm-shifting global company.
Flow's mission is to solve the nation's housing crisis. How? Idk. It involves offering community-centric services in apartment properties to the same remote workforce he once wooed with free beer and a pingpong table. Revolutionary! It seems the goal is to apply WeWork's goals of transforming physical spaces and building community to apartments to solve many of today's housing problems.
The elevator pitch probably sounded great.
At least a16z knows it's a near-impossible task, calling it a seismic shift. Marc Andreessen opposes affordable housing in his wealthy Silicon Valley town. As details of the project emerge, more investors will likely throw ethics and morals out the window to go with the flow, throwing money at a man known for burning through it while building toxic companies, hoping he can bank another fantasy valuation before it all crashes.
Insanity is repeating the same action and expecting a different result. Everyone on the Neumann hype train needs to sober up.
Like WeWork, this venture Won’tWork.
Like before, it'll cause a shitstorm.

Nick Nolan
1 year ago
How to Make $1,037,100 in 4 Months with This Weird Website
One great idea might make you rich.
Imagine having a million-dollar concept in college that made a million.
2005 precisely.
Alex Tew, 21, from Wiltshire, England, created The Million Dollar Homepage in August 2005. The idea is basic but beyond the ordinary, which is why it worked.
Alex built a 1,000,000-pixel webpage.
Each website pixel would cost $1. Since pixels are hard to discern, he sold 10x10 squares for $100.
He'd make a million if all the spots sold.
He may have thought about NFTs and the Metaverse decades ago.
MillionDollarHomepage.com launched in 2005.
Businesses and individuals could buy a website spot and add their logo, website link, and tagline. You bought an ad, but nobody visited the website.
If a few thousand people visited the website, it could drive traffic to your business's site.
Alex promised buyers the website would be up for 5 years, so it was a safe bet.
Alex's friend with a music website was the first to buy real estate on the site. Within two weeks, 4,700 pixels sold, and a tracker showed how many were sold and available.
Word-of-mouth marketing got the press's attention quickly. Everyone loves reading about new ways to make money, so it was a good news story.
By September, over 250,000 pixels had been sold, according to a BBC press release.
Alex and the website gained more media and public attention, so traffic skyrocketed. Two months after the site launched, 1,400 customers bought more than 500,000 pixels.
Businesses bought online real estate. They heard thousands visited the site, so they could get attention cheaply.
Unless you bought a few squares, I'm not sure how many people would notice your ad or click your link.
A sponge website owner emailed Alex:
“We tried Million Dollar Homepage because we were impressed at the level of ingenuity and the sheer simplicity of it. If we’re honest, we didn’t expect too much from it. Now, as a direct result, we are pitching for £18,000 GBP worth of new clients and have seen our site traffic increase over a hundred-fold. We’re even going to have to upgrade our hosting facility! It’s been exceptional.”
Web.archive.org screenshots show how the website changed.
“The idea is to create something of an internet time capsule: a homepage that is unique and permanent. Everything on the internet keeps changing so fast, it will be nice to have something that stays solid and permanent for many years. You can be a part of that!” Alex Tew, 2005
The last 1,000 pixels were sold on January 1, 2006.
By then, the homepage had hundreds of thousands of monthly visitors. Alex put the last space on eBay due to high demand.
MillionDollarWeightLoss.com won the last pixels for $38,100, bringing revenue to $1,037,100 in 4 months.
Many have tried to replicate this website's success. They've all failed.
This idea only worked because no one had seen this website before.
This winner won't be repeated, but it should inspire you to try something new and creative.
Still popular, you could buy one of the linked domains. You can't buy pixels, but you can buy an expired domain.
One link I clicked costs $59,888.
You'd own a piece of internet history if you spent that much on a domain.
Someone bought stablesgallery.co.uk after the domain expired and restored it.
Many of the linked websites have expired or been redirected, but some still link to the original. I couldn't find sponge's website. Can you?
This is a great example of how a simple creative idea can go viral.
Comment on this amazing success story.
You might also like

Sukhad Anand
1 year ago
How Do Discord's Trillions Of Messages Get Indexed?
They depend heavily on open source..
Discord users send billions of messages daily. Users wish to search these messages. How do we index these to search by message keywords?
Let’s find out.
Discord utilizes Elasticsearch. Elasticsearch is a free, open search engine for textual, numerical, geographical, structured, and unstructured data. Apache Lucene powers Elasticsearch.
How does elastic search store data? It stores it as numerous key-value pairs in JSON documents.
How does elastic search index? Elastic search's index is inverted. An inverted index lists every unique word in every page and where it appears.
4. Elasticsearch indexes documents and generates an inverted index to make data searchable in near real-time. The index API adds or updates JSON documents in a given index.
Let's examine how discord uses Elastic Search. Elasticsearch prefers bulk indexing. Discord couldn't index real-time messages. You can't search posted messages. You want outdated messages.
6. Let's check what bulk indexing requires.
1. A temporary queue for incoming communications.
2. Indexer workers that index messages into elastic search.
Discord's queue is Celery. The queue is open-source. Elastic search won't run on a single server. It's clustered. Where should a message go? Where?
8. A shard allocator decides where to put the message. Nevertheless. Shattered? A shard combines elastic search and index on. So, these two form a shard which is used as a unit by discord. The elastic search itself has some shards. But this is different, so don’t get confused.
Now, the final part is service discovery — to discover the elastic search clusters and the hosts within that cluster. This, they do with the help of etcd another open source tool.
A great thing to notice here is that discord relies heavily on open source systems and their base implementations which is very different from a lot of other products.

Ari Joury, PhD
1 year ago
7 ways to turn into a major problem-solver
For some people, the glass is half empty. For others, it’s half full. And for some, the question is, How do I get this glass totally full again?
Problem-solvers are the last group. They're neutral. Pragmatists.
Problems surround them. They fix things instead of judging them. Problem-solvers improve the world wherever they go.
Some fail. Sometimes their good intentions have terrible results. Like when they try to help a grandma cross the road because she can't do it alone but discover she never wanted to.
Most programmers, software engineers, and data scientists solve problems. They use computer code to fix problems they see.
Coding is best done by understanding and solving the problem.
Despite your best intentions, building the wrong solution may have negative consequences. Helping an unwilling grandma cross the road.
How can you improve problem-solving?
1. Examine your presumptions.
Don’t think There’s a grandma, and she’s unable to cross the road. Therefore I must help her over the road. Instead think This grandma looks unable to cross the road. Let’s ask her whether she needs my help to cross it.
Maybe the grandma can’t cross the road alone, but maybe she can. You can’t tell for sure just by looking at her. It’s better to ask.
Maybe the grandma wants to cross the road. But maybe she doesn’t. It’s better to ask!
Building software is similar. Do only I find this website ugly? Who can I consult?
We all have biases, mental shortcuts, and worldviews. They simplify life.
Problem-solving requires questioning all assumptions. They might be wrong!
Think less. Ask more.
Secondly, fully comprehend the issue.
Grandma wants to cross the road? Does she want flowers from the shop across the street?
Understanding the problem advances us two steps. Instead of just watching people and their challenges, try to read their intentions.
Don't ask, How can I help grandma cross the road? Why would this grandma cross the road? What's her goal?
Understand what people want before proposing solutions.
3. Request more information. This is not a scam!
People think great problem solvers solve problems immediately. False!
Problem-solvers study problems. Understanding the problem makes solving it easy.
When you see a grandma struggling to cross the road, you want to grab her elbow and pull her over. However, a good problem solver would ask grandma what she wants. So:
Problem solver: Excuse me, ma’am? Do you wish to get over the road? Grandma: Yes indeed, young man! Thanks for asking. Problem solver: What do you want to do on the other side? Grandma: I want to buy a bouquet of flowers for my dear husband. He loves flowers! I wish the shop wasn’t across this busy road… Problem solver: Which flowers does your husband like best? Grandma: He loves red dahlia. I usually buy about 20 of them. They look so pretty in his vase at the window! Problem solver: I can get those dahlia for you quickly. Go sit on the bench over here while you’re waiting; I’ll be back in five minutes. Grandma: You would do that for me? What a generous young man you are!
A mediocre problem solver would have helped the grandma cross the road, but he might have forgotten that she needs to cross again. She must watch out for cars and protect her flowers on the way back.
A good problem solver realizes that grandma's husband wants 20 red dahlias and completes the task.
4- Rapid and intense brainstorming
Understanding a problem makes solutions easy. However, you may not have all the information needed to solve the problem.
Additionally, retrieving crucial information can be difficult.
You could start a blog. You don't know your readers' interests. You can't ask readers because you don't know who they are.
Brainstorming works here. Set a stopwatch (most smartphones have one) to ring after five minutes. In the remaining time, write down as many topics as possible.
No answer is wrong. Note everything.
Sort these topics later. Programming or data science? What might readers scroll past—are these your socks this morning?
Rank your ideas intuitively and logically. Write Medium stories using the top 35 ideas.
5 - Google it.
Doctor Google may answer this seemingly insignificant question. If you understand your problem, try googling or binging.
Someone has probably had your problem before. The problem-solver may have posted their solution online.
Use others' experiences. If you're social, ask a friend or coworker for help.
6 - Consider it later
Rest your brain.
Reread. Your brain needs rest to function.
Hustle culture encourages working 24/7. It doesn't take a neuroscientist to see that this is mental torture.
Leave an unsolvable problem. Visit friends, take a hot shower, or do whatever you enjoy outside of problem-solving.
Nap.
I get my best ideas in the morning after working on a problem. I couldn't have had these ideas last night.
Sleeping subconsciously. Leave it alone and you may be surprised by the genius it produces.
7 - Learn to live with frustration
There are problems that you’ll never solve.
Mathematicians are world-class problem-solvers. The brightest minds in history have failed to solve many mathematical problems.
A Gordian knot problem can frustrate you. You're smart!
Frustration-haters don't solve problems well. They choose simple problems to avoid frustration.
No. Great problem solvers want to solve a problem but know when to give up.
Frustration initially hurts. You adapt.
Famous last words
If you read this article, you probably solve problems. We've covered many ways to improve, so here's a summary:
Test your presumptions. Is the issue the same for everyone else when you see one? Or are your prejudices and self-judgments misguiding you?
Recognize the issue completely. On the surface, a problem may seem straightforward, but what's really going on? Try to see what the current situation might be building up to by thinking two steps ahead of the current situation.
Request more information. You are no longer a high school student. A two-sentence problem statement is not sufficient to provide a solution. Ask away if you need more details!
Think quickly and thoroughly. In a constrained amount of time, try to write down all your thoughts. All concepts are worthwhile! Later, you can order them.
Google it. There is a purpose for the internet. Use it.
Consider it later at night. A rested mind is more creative. It might seem counterintuitive to leave a problem unresolved. But while you're sleeping, your subconscious will handle the laborious tasks.
Accept annoyance as a normal part of life. Don't give up if you're feeling frustrated. It's a step in the procedure. It's also perfectly acceptable to give up on a problem because there are other, more pressing issues that need to be addressed.
You might feel stupid sometimes, but that just shows that you’re human. You care about the world and you want to make it better.
At the end of the day, that’s all there is to problem solving — making the world a little bit better.
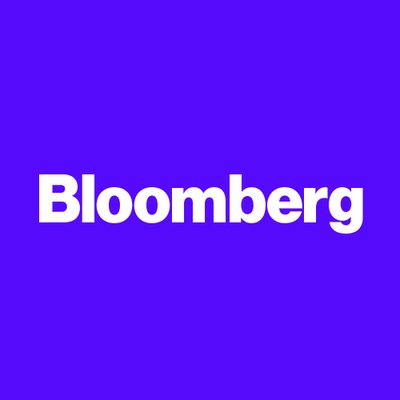
Bloomberg
2 years ago
Expulsion of ten million Ukrainians
According to recent data from two UN agencies, ten million Ukrainians have been displaced.
The International Organization for Migration (IOM) estimates nearly 6.5 million Ukrainians have relocated. Most have fled the war zones around Kyiv and eastern Ukraine, including Dnipro, Zhaporizhzhia, and Kharkiv. Most IDPs have fled to western and central Ukraine.
Since Russia invaded on Feb. 24, 3.6 million people have crossed the border to seek refuge in neighboring countries, according to the latest UN data. While most refugees have fled to Poland and Romania, many have entered Russia.
Internally displaced figures are IOM estimates as of March 19, based on 2,000 telephone interviews with Ukrainians aged 18 and older conducted between March 9-16. The UNHCR compiled the figures for refugees to neighboring countries on March 21 based on official border crossing data and its own estimates. The UNHCR's top-line total is lower than the country totals because Romania and Moldova totals include people crossing between the two countries.
Sources: IOM, UNHCR
According to IOM estimates based on telephone interviews with a representative sample of internally displaced Ukrainians, over 53% of those displaced are women, and over 60% of displaced households have children.