More on Technology

Nikhil Vemu
1 year ago
7 Mac Tips You Never Knew You Needed
Unleash the power of the Option key ⌥
#1 Open a link in the Private tab first.
Previously, if I needed to open a Safari link in a private window, I would:
copied the URL with the right click command,
choose File > New Private Window to open a private window, and
clicked return after pasting the URL.
I've found a more straightforward way.
Right-clicking a link shows this, right?
Hold option (⌥) for:
Click Open Link in New Private Window while holding.
Finished!
#2. Instead of searching for specific characters, try this
You may use unicode for business or school. Most people Google them when they need them.
That is lengthy!
You can type some special characters just by pressing ⌥ and a key.
For instance
• ⌥+2 -> ™ (Trademark)
• ⌥+0 -> ° (Degree)
• ⌥+G -> © (Copyright)
• ⌥+= -> ≠ (Not equal to)
• ⌥+< -> ≤ (Less than or equal to)
• ⌥+> -> ≥ (Greater then or equal to)
• ⌥+/ -> ÷ (Different symbol for division)
#3 Activate Do Not Disturb silently.
Do Not Disturb when sharing my screen is awkward for me (because people may think Im trying to hide some secret notifications).
Here's another method.
Hold ⌥ and click on Time (at the extreme right on the menu-bar).
Now, DND is activated (secretly!). To turn it off, do it again.
Note: This works only for DND focus.
#4. Resize a window starting from its center
Although this is rarely useful, it is still a hidden trick.
When you resize a window, the opposite edge or corner is used as the pivot, right?
However, if you want to resize it with its center as the pivot, hold while doing so.
#5. Yes, Cut-Paste is available on Macs as well (though it is slightly different).
I call it copy-move rather than cut-paste. This is how it works.
Carry it out.
Choose a file (by clicking on it), then copy it (⌘+C).
Go to a new location on your Mac. Do you use ⌘+V to paste it? However, to move it, press ⌘+⌥+V.
This removes the file from its original location and copies it here. And it works exactly like cut-and-paste on Windows.
#6. Instantly expand all folders
Set your Mac's folders to List view.
Assume you have one folder with multiple subfolders, each of which contains multiple files. And you wanted to look at every single file that was over there.
How would you do?
You're used to clicking the ⌄ glyph near the folder and each subfolder to expand them all, right? Instead, hold down ⌥ while clicking ⌄ on the parent folder.
This is what happens next.
Everything expands.
View/Copy a file's path as an added bonus
If you want to see the path of a file in Finder, select it and hold ⌥, and you'll see it at the bottom for a moment.
To copy its path, right-click on the folder and hold down ⌥ to see this
Click on Copy <"folder name"> as Pathname
to do it.
#7 "Save As"
I was irritated by the lack of "Save As" in Pages when I first got a Mac (after 15 years of being a Windows guy).
It was necessary for me to save the file as a new file, in a different location, with a different name, or both.
Unfortunately, I couldn't do it on a Mac.
However, I recently discovered that it appears when you hold ⌥ when in the File menu.
Yay!

Nikhil Vemu
1 year ago
7 Mac Apps That Are Exorbitantly Priced But Totally Worth It
Wish you more bang for your buck
By ‘Cost a Bomb’ I didn’t mean to exaggerate. It’s an idiom that means ‘To be very expensive’. In fact, no app on the planet costs a bomb lol.
So, to the point.
Chronicle
(Freemium. For Pro, $24.99 | Available on Setapp)
You probably have trouble keeping track of dozens of bills and subscriptions each month.
Try Chronicle.
Easy-to-use app
Add payment due dates and receive reminders,
Save payment documentation,
Analyze your spending by season, year, and month.
Observe expenditure trends and create new budgets.
Best of all, Chronicle features an integrated browser for fast payment and logging.
iOS and macOS sync.
SoundSource
($39 for lifetime)
Background Music, a free macOS program, was featured in #6 of this post last month.
It controls per-app volume, stereo balance, and audio over its max level.
Background Music is fully supported. Additionally,
Connect various speakers to various apps (Wow! ),
change the audio sample rate for each app,
To facilitate access, add a floating SoundSource window.
Use its blocks in Shortcuts app,
On the menu bar, include meters for output/input devices and running programs.
PixelSnap
($39 for lifetime | Available on Setapp)
This software is heaven for UI designers.
It aids you.
quickly calculate screen distances (in pixels) ,
Drag an area around an object to determine its borders,
Measure the distances between the additional guides,
screenshots should be pixel-perfect.
What’s more.
You can
Adapt your tolerance for items with poor contrast and shadows.
Use your Touch Bar to perform important tasks, if you have one.
Mate Translation
($3.99 a month / $29.99 a year | Available on Setapp)
Mate Translate resembles a roided-up version of BarTranslate, which I wrote about in #1 of this piece last month.
If you translate often, utilize Mate Translate on macOS and Safari.
I'm really vocal about it.
It stays on the menu bar, and is accessible with a click or ⌥+shift+T hotkey.
It lets you
Translate in 103 different languages,
To translate text, double-click or right-click on it.
Totally translate websites. Additionally, Netflix subtitles,
Listen to their pronunciation to see how close it is to human.
iPhone and Mac sync Mate-ing history.
Swish
($16 for lifetime | Available on Setapp)
Swish is awesome!
Swipe, squeeze, tap, and hold movements organize chaotic desktop windows. Swish operates with mouse and trackpad.
Some gestures:
• Pinch Once: Close an app
• Pinch Twice: Quit an app
• Swipe down once: Minimise an app
• Pinch Out: Enter fullscreen mode
• Tap, Hold, & Swipe: Arrange apps in grids
and many more...
After getting acquainted to the movements, your multitasking will improve.
Unite
($24.99 for lifetime | Available on Setapp)
It turns webapps into macOS apps. The end.
Unite's functionality is a million times better.
Provide extensive customization (incl. its icon, light and dark modes)
make menu bar applications,
Get badges for web notifications and automatically refresh websites,
Replace any dock icon in the window with it (Wow!) by selecting that portion of the window.
Use PiP (Picture-in-Picture) on video sites that support it.
Delete advertising,
Throughout macOS, use floating windows
and many more…
I feel $24.99 one-off for this tool is a great deal, considering all these features. What do you think?
CleanShot X
(Basic: $29 one-off. Pro: $8/month | Available on Setapp)
CleanShot X can achieve things the macOS screenshot tool cannot. Complete screenshot toolkit.
CleanShot X, like Pixel Snap 2 (#3), is fantastic.
Allows
Scroll to capture a long page,
screen recording,
With webcam on,
• With mic and system audio,
• Highlighting mouse clicks and hotkeys.
Maintain floating screenshots for reference
While capturing, conceal desktop icons and notifications.
Recognize text in screenshots (OCR),
You may upload and share screenshots using the built-in cloud.
These are just 6 in 50+ features, and you’re already saying Wow!

VIP Graphics
2 years ago
Leaked pitch deck for Metas' new influencer-focused live-streaming service
As part of Meta's endeavor to establish an interactive live-streaming platform, the company is testing with influencers.
The NPE (new product experimentation team) has been testing Super since late 2020.
Bloomberg defined Super as a Cameo-inspired FaceTime-like gadget in 2020. The tool has evolved into a Twitch-like live streaming application.
Less than 100 creators have utilized Super: Creators can request access on Meta's website. Super isn't an Instagram, Facebook, or Meta extension.
“It’s a standalone project,” the spokesperson said about Super. “Right now, it’s web only. They have been testing it very quietly for about two years. The end goal [of NPE projects] is ultimately creating the next standalone project that could be part of the Meta family of products.” The spokesperson said the outreach this week was part of a drive to get more creators to test Super.
A 2021 pitch deck from Super reveals the inner workings of Meta.
The deck gathered feedback on possible sponsorship models, with mockups of brand deals & features. Meta reportedly paid creators $200 to $3,000 to test Super for 30 minutes.
Meta's pitch deck for Super live streaming was leaked.
What were the slides in the pitch deck for Metas Super?
Embed not supported: see full deck & article here →
View examples of Meta's pitch deck for Super:
Product Slides, first
The pitch deck begins with Super's mission:
Super is a Facebook-incubated platform which helps content creators connect with their fans digitally, and for super fans to meet and support their favorite creators. In the spirit of Late Night talk shows, we feature creators (“Superstars”), who are guests at a live, hosted conversation moderated by a Host.
This slide (and most of the deck) is text-heavy, with few icons, bullets, and illustrations to break up the content. Super's online app status (which requires no download or installation) might be used as a callout (rather than paragraph-form).
Meta's Super platform focuses on brand sponsorships and native placements, as shown in the slide above.
One of our theses is the idea that creators should benefit monetarily from their Super experiences, and we believe that offering a menu of different monetization strategies will enable the right experience for each creator. Our current focus is exploring sponsorship opportunities for creators, to better understand what types of sponsor placements will facilitate the best experience for all Super customers (viewers, creators, and advertisers).
Colorful mockups help bring Metas vision for Super to life.
2. Slide Features
Super's pitch deck focuses on the platform's features. The deck covers pre-show, pre-roll, and post-event for a Sponsored Experience.
Pre-show: active 30 minutes before the show's start
Pre-roll: Play a 15-minute commercial for the sponsor before the event (auto-plays once)
Meet and Greet: This event can have a branding, such as Meet & Greet presented by [Snickers]
Super Selfies: Makers and followers get a digital souvenir to post on social media.
Post-Event: Possibility to draw viewers' attention to sponsored content/links during the after-show
Almost every screen displays the Sponsor logo, link, and/or branded background. Viewers can watch sponsor video while waiting for the event to start.
Slide 3: Business Model
Meta's presentation for Super is incomplete without numbers. Super's first slide outlines the creator, sponsor, and Super's obligations. Super does not charge creators any fees or commissions on sponsorship earnings.
How to make a great pitch deck
We hope you can use the Super pitch deck to improve your business. Bestpitchdeck.com/super-meta is a bookmarkable link.
You can also use one of our expert-designed templates to generate a pitch deck.
Our team has helped close $100M+ in agreements and funding for premier companies and VC firms. Use our presentation templates, one-pagers, or financial models to launch your pitch.
Every pitch must be audience-specific. Our team has prepared pitch decks for various sectors and fundraising phases.
Pitch Deck Software VIP.graphics produced a popular SaaS & Software Pitch Deck based on decks that closed millions in transactions & investments for orgs of all sizes, from high-growth startups to Fortune 100 enterprises. This easy-to-customize PowerPoint template includes ready-made features and key slides for your software firm.
Accelerator Pitch Deck The Accelerator Pitch Deck template is for early-stage founders seeking funding from pitch contests, accelerators, incubators, angels, or VC companies. Winning a pitch contest or getting into a top accelerator demands a strategic investor pitch.
Pitch Deck Template Series Startup and founder pitch deck template: Workable, smart slides. This pitch deck template is for companies, entrepreneurs, and founders raising seed or Series A finance.
M&A Pitch Deck Perfect Pitch Deck is a template for later-stage enterprises engaging more sophisticated conversations like M&A, late-stage investment (Series C+), or partnerships & funding. Our team prepared this presentation to help creators confidently pitch to investment banks, PE firms, and hedge funds (and vice versa).
Browse our growing variety of industry-specific pitch decks.
You might also like
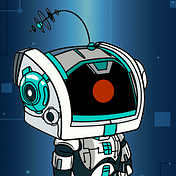
CyberPunkMetalHead
1 year ago
I talked to ChatGPT about the adoption of cryptocurrencies.
OpenAI's ChatGPT is an amazing AI-powered chatbot. It can write poetry, fiction, and marketing ideas. I was impressed how well ChatGPT followed the interview, albeit there were a few classics “I’m afraid I can’t do that”.
Full interview below.
Me: I'm your interviewer. We'll discuss crypto. Thanks for being interviewed. What do you think about cryptocurrency?
CGPT: Cryptocurrency is an innovative technology that could transform money and financial activities. It might boost the efficiency and security of financial institutions and let consumers deal directly without banks. Cryptocurrency is still a new and untested technology with many unknowns and hurdles before it can be broadly accepted.
Me: Over 22,000 cryptocurrencies exist, and many have little use. We need how much cryptocurrencies?
CGPT: The appropriate quantity will rely on a multitude of factors, including each cryptocurrency's use cases, adoption rate, and market rivalry. Only a few cryptocurrencies will likely receive widespread adoption and be successful. Many existing cryptocurrencies may not survive since they can't deliver new or valued solutions or compete with more established cryptocurrencies.
Me: Will cryptocurrencies grow? What's next for worldwide adoption?
CGPT: It's hard to forecast the future of cryptocurrencies because the acceptance of new technologies is unpredictable. I believe cryptocurrencies will expand and acquire popularity in the future years. Regulatory ambiguity, security concerns, and scalability issues must be overcome for this to happen. Cryptocurrency companies and organizations must engage together with governments and authorities to address these obstacles and establish a growth-friendly climate. Continued innovation and improvement of the technology, including public education and awareness, will drive acceptance.
Me: Do bear markets affect bitcoin adoption?
CGPT: On the one hand, a bear market might induce uncertainty and hesitation among potential consumers. Bear markets can present opportunities for long-term investors who can acquire cryptocurrencies at cheaper prices and are more likely to hang onto their assets. A bear market can also test the tenacity and power of the underlying technology and community, which can boost long-term confidence and adoption. A bear market might have profound and nuanced effects on bitcoin adoption.
Me: What are some likely outcomes if Bitcoin reaches $100,000 by next year?
CGPT: If Bitcoin saw rapid growth, it would undoubtedly have repercussions for the cryptocurrency market and economy. Possible scenarios include increased public interest and awareness of cryptocurrency, a surge in demand for Bitcoin and other cryptocurrencies, and a growth in the cryptocurrency industry's market value. By shifting their focus and cash to the cryptocurrency market, investors and traders may also affect other asset classes, such as traditional equities and commodities. Governments and financial organizations may focus more on the cryptocurrency sector as they try to grasp Bitcoin's rapid rise.
Me: Will other countries accept crypto as currency?
CGPT: It's hard to say if additional governments will adopt cryptocurrencies as legal tender. Some countries have recognized the promise of bitcoin and are exploring its use, while others are cautious. Adoption of bitcoin as legal cash may vary by country, based on financial infrastructure, rules, and political atmosphere. As cryptocurrency technology evolves and becomes more accepted, more governments may embrace it as legal tender.
Me: Final question: will the recent cryptocurrency hacks affect long term adoption?
CGPT: Hacks might tarnish cryptocurrency's reputation and public faith in its security, the technology underpinning it is continually improving. Many cryptocurrency projects have developed strong security mechanisms to prevent hacks and other dangers, and these safeguards will undoubtedly improve over time. The decentralized nature of cryptocurrency makes it more resistant to attacks. Hacks and other security breaches are a concern, but they may not affect cryptocurrency adoption.

Pat Vieljeux
2 years ago
In 5 minutes, you can tell if a startup will succeed.
Or the “lie to me” method.
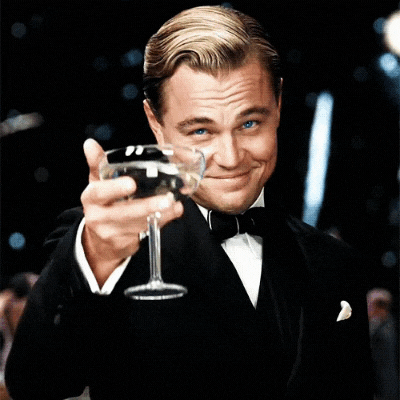
I can predict a startup's success in minutes.
Just interview its founder.
Ask "why?"
I question "why" till I sense him.
I need to feel the person I have in front of me. I need to know if he or she can deliver. Startups aren't easy. Without abilities, a brilliant idea will fail.
Good entrepreneurs have these qualities: He's a leader, determined, and resilient.
For me, they can be split in two categories.
The first entrepreneur aspires to live meaningfully. The second wants to get rich. The second is communicative. He wants to wow the crowd. He's motivated by the thought of one day sailing a boat past palm trees and sunny beaches.
What drives the first entrepreneur is evident in his speech, face, and voice. He will not speak about his product. He's (nearly) uninterested. He's not selling anything. He's not a salesman. He wants to succeed. The product is his fuel.
He'll explain his decision. He'll share his motivations. His desire. And he'll use meaningful words.
Paul Ekman has shown that face expressions aren't cultural. His study influenced the American TV series "lie to me" about body language and speech.
Passionate entrepreneurs are obvious. It's palpable. Faking passion is tough. Someone who wants your favor and money will expose his actual motives through his expressions and language.
The good liar will be able to fool you for a while, but not for long if you pay attention to his body language and how he expresses himself.
And also, if you look at his business plan.
His business plan reveals his goals. Read between the lines.
Entrepreneur 1 will focus on his "why", whereas Entrepreneur 2 will focus on the "how".
Entrepreneur 1 will develop a vision-driven culture.
The second, on the other hand, will focus on his EBITDA.
Why is the culture so critical? Because it will allow entrepreneur 1 to develop a solid team that can tackle his problems and trials. His team's "why" will keep them together in tough times.
"Give me a terrific start-up team with a mediocre idea over a weak one any day." Because a great team knows when to pivot and trusts each other. Weak teams fail.” — Bernhard Schroeder
Closings thoughts
Every VC must ask Why. Entrepreneur's motivations. This "why" will create the team's culture. This culture will help the team adjust to any setback.
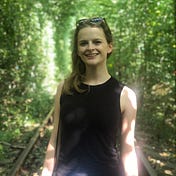
Claire Berehova
2 years ago
There’s no manual for that
Kyiv oblast in springtime. Photo by author. |
We’ve been receiving since the war began text messages from the State Emergency Service of Ukraine every few days. They’ve contained information on how to comfort a child and what to do in case of a water outage.
But a question that I struggle to suppress irks within me: How would we know if there really was a threat coming our away? So how can I happily disregard an air raid siren and continue singing to my three-month-old son when I feel like a World War II film became reality? There’s no manual for that.
Along with the anxiety, there’s the guilt that always seems to appear alongside dinner we’re fortunate to still have each evening while brave Ukrainian soldiers are facing serious food insecurity. There’s no manual for how to deal with this guilt.
When it comes to the enemy, there is no manual for how to react to the news of Russian casualties. Every dead Russian soldier weakens Putin, but I also know that many of these men had wives and girlfriends who are now living a nightmare.
So, I felt like I had to start writing my own manual.
The anxiety around the air raid siren? Only with time does it get easier to ignore it, but never completely.
The guilt? All we can do is pray.
That inner conflict? As Russia continues to stun the world with its war crimes, my emotions get less gray — I have to get used to accommodating absurd levels of hatred.
Sadness? It feels a bit more manageable when we laugh, and a little alcohol helps (as it usually does).
Cabin fever? Step outside in the yard when possible. At least the sunshine is becoming more fervent with spring approaching.
Slava Ukraini. Heroyam slava. (Glory to Ukraine. Glory to the heroes.)