More on Technology

Christianlauer
2 years ago
Looker Studio Pro is now generally available, according to Google.
Great News about the new Google Business Intelligence Solution
Google has renamed Data Studio to Looker Studio and Looker Studio Pro.
Now, Google releases Looker Studio Pro. Similar to the move from Data Studio to Looker Studio, Looker Studio Pro is basically what Looker was previously, but both solutions will merge. Google says the Pro edition will acquire new enterprise management features, team collaboration capabilities, and SLAs.
In addition to Google's announcements and sales methods, additional features include:
Looker Studio assets can now have organizational ownership. Customers can link Looker Studio to a Google Cloud project and migrate existing assets once. This provides:
Your users' created Looker Studio assets are all kept in a Google Cloud project.
When the users who own assets leave your organization, the assets won't be removed.
Using IAM, you may provide each Looker Studio asset in your company project-level permissions.
Other Cloud services can access Looker Studio assets that are owned by a Google Cloud project.
Looker Studio Pro clients may now manage report and data source access at scale using team workspaces.
Google announcing these features for the pro version is fascinating. Both products will likely converge, but Google may only release many features in the premium version in the future. Microsoft with Power BI and its free and premium variants already achieves this.
Sources and Further Readings
Google, Release Notes (2022)
Google, Looker (2022)
Colin Faife
2 years ago
The brand-new USB Rubber Ducky is much riskier than before.
The brand-new USB Rubber Ducky is much riskier than before.
With its own programming language, the well-liked hacking tool may now pwn you.
With a vengeance, the USB Rubber Ducky is back.
This year's Def Con hacking conference saw the release of a new version of the well-liked hacking tool, and its author, Darren Kitchen, was on hand to explain it. We put a few of the new features to the test and discovered that the most recent version is riskier than ever.
WHAT IS IT?
The USB Rubber Ducky seems to the untrained eye to be an ordinary USB flash drive. However, when you connect it to a computer, the computer recognizes it as a USB keyboard and will accept keystroke commands from the device exactly like a person would type them in.
Kitchen explained to me, "It takes use of the trust model built in, where computers have been taught to trust a human, in that anything it types is trusted to the same degree as the user is trusted. And a computer is aware that clicks and keystrokes are how people generally connect with it.
Over ten years ago, the first Rubber Ducky was published, quickly becoming a hacker favorite (it was even featured in a Mr. Robot scene). Since then, there have been a number of small upgrades, but the most recent Rubber Ducky takes a giant step ahead with a number of new features that significantly increase its flexibility and capability.
WHERE IS ITS USE?
The options are nearly unlimited with the proper strategy.
The Rubber Ducky has already been used to launch attacks including making a phony Windows pop-up window to collect a user's login information or tricking Chrome into sending all saved passwords to an attacker's web server. However, these attacks lacked the adaptability to operate across platforms and had to be specifically designed for particular operating systems and software versions.
The nuances of DuckyScript 3.0 are described in a new manual.
The most recent Rubber Ducky seeks to get around these restrictions. The DuckyScript programming language, which is used to construct the commands that the Rubber Ducky will enter into a target machine, receives a significant improvement with it. DuckyScript 3.0 is a feature-rich language that allows users to write functions, store variables, and apply logic flow controls, in contrast to earlier versions that were primarily limited to scripting keystroke sequences (i.e., if this... then that).
This implies that, for instance, the new Ducky can check to see if it is hooked into a Windows or Mac computer and then conditionally run code specific to each one, or it can disable itself if it has been attached to the incorrect target. In order to provide a more human effect, it can also generate pseudorandom numbers and utilize them to add a configurable delay between keystrokes.
The ability to steal data from a target computer by encoding it in binary code and transferring it through the signals intended to instruct a keyboard when the CapsLock or NumLock LEDs should light up is perhaps its most astounding feature. By using this technique, a hacker may plug it in for a brief period of time, excuse themselves by saying, "Sorry, I think that USB drive is faulty," and then take it away with all the credentials stored on it.
HOW SERIOUS IS THE RISK?
In other words, it may be a significant one, but because physical device access is required, the majority of people aren't at risk of being a target.
The 500 or so new Rubber Duckies that Hak5 brought to Def Con, according to Kitchen, were his company's most popular item at the convention, and they were all gone on the first day. It's safe to suppose that hundreds of hackers already possess one, and demand is likely to persist for some time.
Additionally, it has an online development toolkit that can be used to create attack payloads, compile them, and then load them onto the target device. A "payload hub" part of the website makes it simple for hackers to share what they've generated, and the Hak5 Discord is also busy with conversation and helpful advice. This makes it simple for users of the product to connect with a larger community.
It's too expensive for most individuals to distribute in volume, so unless your favorite cafe is renowned for being a hangout among vulnerable targets, it's doubtful that someone will leave a few of them there. To that end, if you intend to plug in a USB device that you discovered outside in a public area, pause to consider your decision.
WOULD IT WORK FOR ME?
Although the device is quite straightforward to use, there are a few things that could cause you trouble if you have no prior expertise writing or debugging code. For a while, during testing on a Mac, I was unable to get the Ducky to press the F4 key to activate the launchpad, but after forcing it to identify itself using an alternative Apple keyboard device ID, the problem was resolved.
From there, I was able to create a script that, when the Ducky was plugged in, would instantly run Chrome, open a new browser tab, and then immediately close it once more without requiring any action from the laptop user. Not bad for only a few hours of testing, and something that could be readily changed to perform duties other than reading technology news.

Duane Michael
2 years ago
Don't Fall Behind: 7 Subjects You Must Understand to Keep Up with Technology
As technology develops, you should stay up to date
You don't want to fall behind, do you? This post covers 7 tech-related things you should know.
You'll learn how to operate your computer (and other electronic devices) like an expert and how to leverage the Internet and social media to create your brand and business. Read on to stay relevant in today's tech-driven environment.
You must learn how to code.
Future-language is coding. It's how we and computers talk. Learn coding to keep ahead.
Try Codecademy or Code School. There are also numerous free courses like Coursera or Udacity, but they take a long time and aren't necessarily self-paced, so it can be challenging to find the time.
Artificial intelligence (AI) will transform all jobs.
Our skillsets must adapt with technology. AI is a must-know topic. AI will revolutionize every employment due to advances in machine learning.
Here are seven AI subjects you must know.
What is artificial intelligence?
How does artificial intelligence work?
What are some examples of AI applications?
How can I use artificial intelligence in my day-to-day life?
What jobs have a high chance of being replaced by artificial intelligence and how can I prepare for this?
Can machines replace humans? What would happen if they did?
How can we manage the social impact of artificial intelligence and automation on human society and individual people?
Blockchain Is Changing the Future
Few of us know how Bitcoin and blockchain technology function or what impact they will have on our lives. Blockchain offers safe, transparent, tamper-proof transactions.
It may alter everything from business to voting. Seven must-know blockchain topics:
Describe blockchain.
How does the blockchain function?
What advantages does blockchain offer?
What possible uses for blockchain are there?
What are the dangers of blockchain technology?
What are my options for using blockchain technology?
What does blockchain technology's future hold?
Cryptocurrencies are here to stay
Cryptocurrencies employ cryptography to safeguard transactions and manage unit creation. Decentralized cryptocurrencies aren't controlled by governments or financial institutions.
Bitcoin, the first cryptocurrency, was launched in 2009. Cryptocurrencies can be bought and sold on decentralized exchanges.
Bitcoin is here to stay.
Bitcoin isn't a fad, despite what some say. Since 2009, Bitcoin's popularity has grown. Bitcoin is worth learning about now. Since 2009, Bitcoin has developed steadily.
With other cryptocurrencies emerging, many people are wondering if Bitcoin still has a bright future. Curiosity is natural. Millions of individuals hope their Bitcoin investments will pay off since they're popular now.
Thankfully, they will. Bitcoin is still running strong a decade after its birth. Here's why.
The Internet of Things (IoT) is no longer just a trendy term.
IoT consists of internet-connected physical items. These items can share data. IoT is young but developing fast.
20 billion IoT-connected devices are expected by 2023. So much data! All IT teams must keep up with quickly expanding technologies. Four must-know IoT topics:
Recognize the fundamentals: Priorities first! Before diving into more technical lingo, you should have a fundamental understanding of what an IoT system is. Before exploring how something works, it's crucial to understand what you're working with.
Recognize Security: Security does not stand still, even as technology advances at a dizzying pace. As IT professionals, it is our duty to be aware of the ways in which our systems are susceptible to intrusion and to ensure that the necessary precautions are taken to protect them.
Be able to discuss cloud computing: The cloud has seen various modifications over the past several years once again. The use of cloud computing is also continually changing. Knowing what kind of cloud computing your firm or clients utilize will enable you to make the appropriate recommendations.
Bring Your Own Device (BYOD)/Mobile Device Management (MDM) is a topic worth discussing (MDM). The ability of BYOD and MDM rules to lower expenses while boosting productivity among employees who use these services responsibly is a major factor in their continued growth in popularity.
IoT Security is key
As more gadgets connect, they must be secure. IoT security includes securing devices and encrypting data. Seven IoT security must-knows:
fundamental security ideas
Authorization and identification
Cryptography
electronic certificates
electronic signatures
Private key encryption
Public key encryption
Final Thoughts
With so much going on in the globe, it can be hard to stay up with technology. We've produced a list of seven tech must-knows.
You might also like

Bart Krawczyk
2 years ago
Understanding several Value Proposition kinds will help you create better goods.
Fixing problems isn't enough.
Numerous articles and how-to guides on value propositions focus on fixing consumer concerns.
Contrary to popular opinion, addressing customer pain rarely suffices. Win your market category too.
Core Value Statement
Value proposition usually means a product's main value.
Its how your product solves client problems. The product's core.
Answering these questions creates a relevant core value proposition:
What tasks is your customer trying to complete? (Jobs for clients)
How much discomfort do they feel while they perform this? (pains)
What would they like to see improved or changed? (gains)
After that, you create products and services that alleviate those pains and give value to clients.
Value Proposition by Category
Your product belongs to a market category and must follow its regulations, regardless of its value proposition.
Creating a new market category is challenging. Fitting into customers' product perceptions is usually better than trying to change them.
New product users simplify market categories. Products are labeled.
Your product will likely be associated with a collection of products people already use.
Example: IT experts will use your communication and management app.
If your target clients think it's an advanced mail software, they'll compare it to others and expect things like:
comprehensive calendar
spam detectors
adequate storage space
list of contacts
etc.
If your target users view your product as a task management app, things change. You can survive without a contact list, but not status management.
Find out what your customers compare your product to and if it fits your value offer. If so, adapt your product plan to dominate this market. If not, try different value propositions and messaging to put the product in the right context.
Finished Value Proposition
A comprehensive value proposition is when your solution addresses user problems and wins its market category.
Addressing simply the primary value proposition may produce a valuable and original product, but it may struggle to cross the chasm into the mainstream market. Meeting expectations is easier than changing views.
Without a unique value proposition, you will drown in the red sea of competition.
To conclude:
Find out who your target consumer is and what their demands and problems are.
To meet these needs, develop and test a primary value proposition.
Speak with your most devoted customers. Recognize the alternatives they use to compare you against and the market segment they place you in.
Recognize the requirements and expectations of the market category.
To meet or surpass category standards, modify your goods.
Great products solve client problems and win their category.

Victoria Kurichenko
2 years ago
Updates From Google For Content Producers What You Should Know Is This
People-first update.
Every Google upgrade causes website owners to panic.
Some have just recovered from previous algorithm tweaks and resumed content development.
If you follow Google's Webmaster rules, you shouldn't fear its adjustments.
Everyone has a view of them. Miscommunication and confusion result.
Now, for some (hopefully) exciting news.
Google tweeted on August 18, 2022 about a fresh content update.
This change is another Google effort to remove low-quality, repetitive, and AI-generated content.
The algorithm generates and analyzes search results, not humans.
Google spends a lot to teach its algorithm what searchers want. Intent isn't always clear.
Google's content update aims to:
“… ensure people see more original, helpful content written by people, for people, in search results.”
Isn't it a noble goal?
However, what does it mean for content creators and website owners?
How can you ensure you’re creating content that will be successful after the updates roll out?
Let's first define people-first content.
What does "people-first-content" mean?
If asked, I'd say information written to answer queries and solve problems.
Like others, I read it from the term.
Content creators and marketers disagree. They need more information to follow recommendations.
Google gives explicit instructions for creating people-first content.
According to Google, if you answer yes to the following questions, you have a people-first attitude.
Do you have customers who might find your content useful if they contacted you directly?
Does your content show the breadth of your knowledge?
Do you have a niche or a focus for your website?
After reading your content, will readers learn something new to aid them in achieving their goals?
Are readers happy after reading your content?
Have you been adhering to Google's fundamental updates and product reviews?
As an SEO writer, I'm not scared.
I’ve been following these rules consciously while creating content for my website. That’s why it’s been steadily growing despite me publishing just one or two stories a month.
If you avoid AI-generated text and redundant, shallow material, your website won't suffer.
If you use unscrupulous methods to boost your website's traffic, including link buying or keyword stuffing, stop. Google is getting smarter and will find and punish your site eventually.
For those who say, “SEO is no longer working,” I dedicated the whole paragraph below.
This does not imply that SEO is obsolete.
Google:
“People-first content creators focus on creating satisfying content, while also utilizing SEO best practices to bring searchers additional value.”
The official helpful content update page lists two people-first content components:
meeting user needs
best practices for SEO
Always read official guidelines, not unsolicited suggestions.
SEO will work till search engines die.
How to use the update
Google said the changes will arrive in August 2022.
They pledged to post updates on Google's search ranking updates page.
Google also tweets this info. If you haven't followed it already, I recommend it.
Ranking adjustments could take two weeks and will affect English searches internationally initially.
Google affirmed plans to extend to other languages.
If you own a website, monitor your rankings and traffic to see if it's affected.
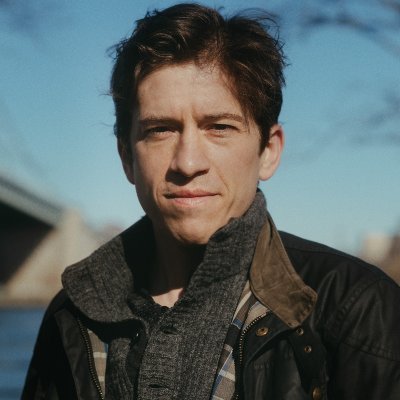
Max Chafkin
2 years ago
Elon Musk Bets $44 Billion on Free Speech's Future
Musk’s purchase of Twitter has sealed his bond with the American right—whether the platform’s left-leaning employees and users like it or not.
Elon Musk's pursuit of Twitter Inc. began earlier this month as a joke. It started slowly, then spiraled out of control, culminating on April 25 with the world's richest man agreeing to spend $44 billion on one of the most politically significant technology companies ever. There have been bigger financial acquisitions, but Twitter's significance has always outpaced its balance sheet. This is a unique Silicon Valley deal.
To recap: Musk announced in early April that he had bought a stake in Twitter, citing the company's alleged suppression of free speech. His complaints were vague, relying heavily on the dog whistles of the ultra-right. A week later, he announced he'd buy the company for $54.20 per share, four days after initially pledging to join Twitter's board. Twitter's directors noticed the 420 reference as well, and responded with a “shareholder rights” plan (i.e., a poison pill) that included a 420 joke.
Musk - Patrick Pleul/Getty Images
No one knew if the bid was genuine. Musk's Twitter plans seemed implausible or insincere. In a tweet, he referred to automated accounts that use his name to promote cryptocurrency. He enraged his prospective employees by suggesting that Twitter's San Francisco headquarters be turned into a homeless shelter, renaming the company Titter, and expressing solidarity with his growing conservative fan base. “The woke mind virus is making Netflix unwatchable,” he tweeted on April 19.
But Musk got funding, and after a frantic weekend of negotiations, Twitter said yes. Unlike most buyouts, Musk will personally fund the deal, putting up up to $21 billion in cash and borrowing another $12.5 billion against his Tesla stock.
Free Speech and Partisanship
Percentage of respondents who agree with the following
The deal is expected to replatform accounts that were banned by Twitter for harassing others, spreading misinformation, or inciting violence, such as former President Donald Trump's account. As a result, Musk is at odds with his own left-leaning employees, users, and advertisers, who would prefer more content moderation rather than less.
Dorsey - Photographer: Joe Raedle/Getty Images
Previously, the company's leadership had similar issues. Founder Jack Dorsey stepped down last year amid concerns about slowing growth and product development, as well as his dual role as CEO of payments processor Block Inc. Compared to Musk, a father of seven who already runs four companies (besides Tesla and SpaceX), Dorsey is laser-focused.
Musk's motivation to buy Twitter may be political. Affirming the American far right with $44 billion spent on “free speech” Right-wing activists have promoted a series of competing upstart Twitter competitors—Parler, Gettr, and Trump's own effort, Truth Social—since Trump was banned from major social media platforms for encouraging rioters at the US Capitol on Jan. 6, 2021. But Musk can give them a social network with lax content moderation and a real user base. Trump said he wouldn't return to Twitter after the deal was announced, but he wouldn't be the first to do so.
Trump - Eli Hiller/Bloomberg
Conservative activists and lawmakers are already ecstatic. “A great day for free speech in America,” said Missouri Republican Josh Hawley. The day the deal was announced, Tucker Carlson opened his nightly Fox show with a 10-minute laudatory monologue. “The single biggest political development since Donald Trump's election in 2016,” he gushed over Musk.
But Musk's supporters and detractors misunderstand how much his business interests influence his political ideology. He marketed Tesla's cars as carbon-saving machines that were faster and cooler than gas-powered luxury cars during George W. Bush's presidency. Musk gained a huge following among wealthy environmentalists who reserved hundreds of thousands of Tesla sedans years before they were made during Barack Obama's presidency. Musk in the Trump era advocated for a carbon tax, but he also fought local officials (and his own workers) over Covid rules that slowed the reopening of his Bay Area factory.
Teslas at the Las Vegas Convention Center Loop Central Station in April 2021. The Las Vegas Convention Center Loop was Musk's first commercial project. Ethan Miller/Getty Images
Musk's rightward shift matched the rise of the nationalist-populist right and the desire to serve a growing EV market. In 2019, he unveiled the Cybertruck, a Tesla pickup, and in 2018, he announced plans to manufacture it at a new plant outside Austin. In 2021, he decided to move Tesla's headquarters there, citing California's "land of over-regulation." After Ford and General Motors beat him to the electric truck market, Musk reframed Tesla as a company for pickup-driving dudes.
Similarly, his purchase of Twitter will be entwined with his other business interests. Tesla has a factory in China and is friendly with Beijing. This could be seen as a conflict of interest when Musk's Twitter decides how to treat Chinese-backed disinformation, as Amazon.com Inc. founder Jeff Bezos noted.
Musk has focused on Twitter's product and social impact, but the company's biggest challenges are financial: Either increase cash flow or cut costs to comfortably service his new debt. Even if Musk can't do that, he can still benefit from the deal. He has recently used the increased attention to promote other business interests: Boring has hyperloops and Neuralink brain implants on the way, Musk tweeted. Remember Tesla's long-promised robotaxis!
Musk may be comfortable saying he has no expectation of profit because it benefits his other businesses. At the TED conference on April 14, Musk insisted that his interest in Twitter was solely charitable. “I don't care about money.”
The rockets and weed jokes make it easy to see Musk as unique—and his crazy buyout will undoubtedly add to that narrative. However, he is a megabillionaire who is risking a small amount of money (approximately 13% of his net worth) to gain potentially enormous influence. Musk makes everything seem new, but this is a rehash of an old media story.